Welcome to our research lab, where we strive to understand the genetic basis of complex traits and diseases. Using a combination of statistical methods and genomic data, we are exploring the intricate genetic landscape that shapes these traits and diseases.
Our research also extends beyond simply exploring associations between genetic variants and disease-related or other phenotypes; we are keenly interested in determining the biological mechanisms through which those associations occur. To achieve this goal, we use a wide range of molecular phenotypes such as RNA sequencing, proteomics, chromatin profiling and methylomic profiling – allowing us to get an even deeper look into how genetic variation impacts human biology.
Functional Genomics
Multi-omic and complex trait genetics
Only ~1/3 of GWAS variants colocalize with steady-state cis- expression QTLs, and the majority GWAS variants have unexplained regulatory mechanisms. To interpret and understand trait-associated variants, we work with different types of molecular phenotypes (RNA-seq, ChIP-seq, ATAC-seq, CUT&TAG etc) and develop methods to identify genetic effects of these molecular phenotypes. We develop powerful statistical tools to identify genetic effects associated with these molecular phenotypes and uncover disease underlying molecular mechanisms.
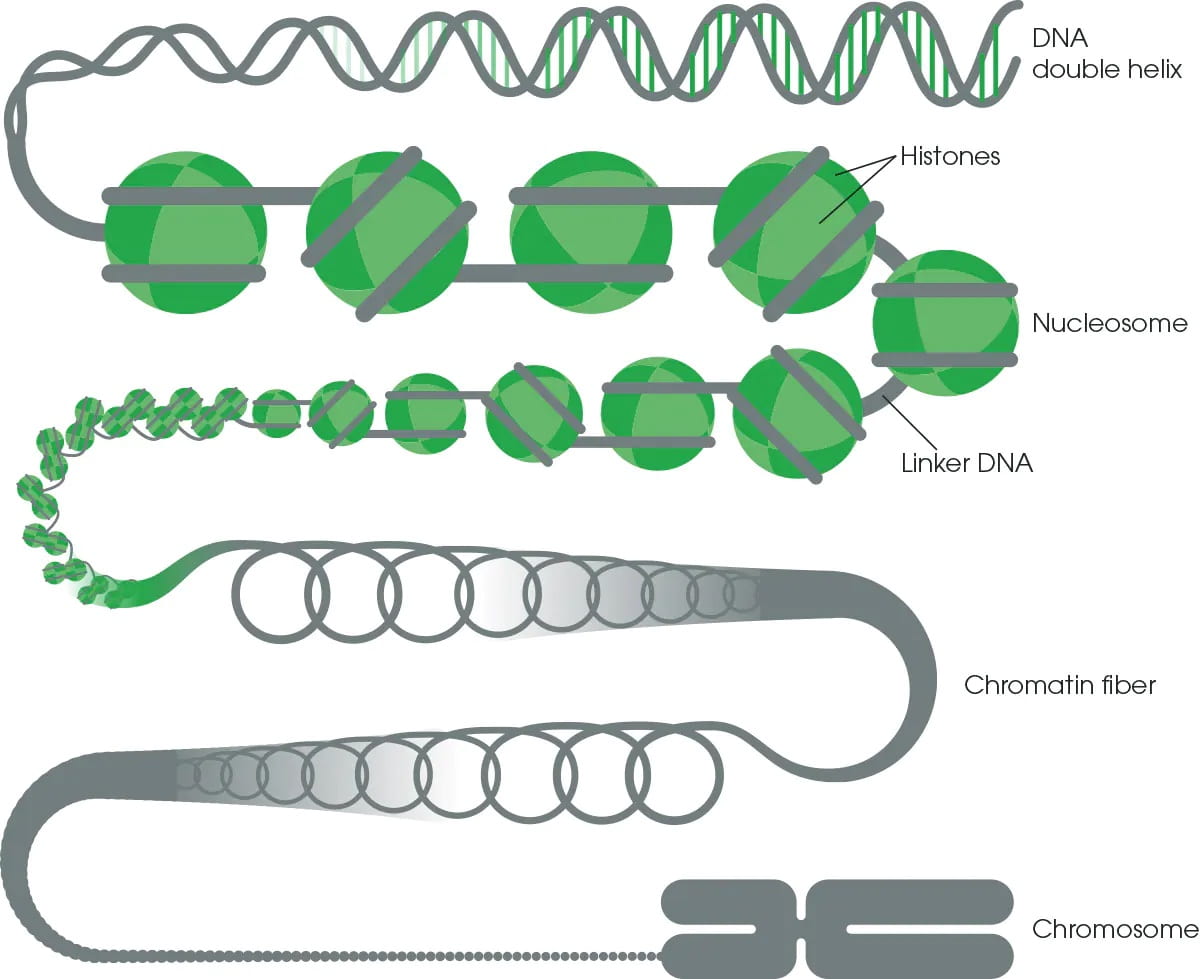
Statistical methods in single-cell sequencing datasets
Sequencing technologies in single cells (eg. scRNAseq, scATACseq etc) are poised to replace bulk tissues, as they allow dissecting molecular processes and the genetic control of these processes at single-cell resolution. Our lab combines robust statistical methods and single-cell data to gain insights into disease biology.
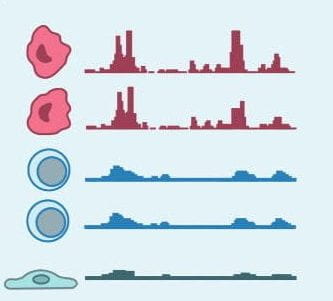
Trans gene regulation
Previously, we proposed that trans-genetic effects manifested in gene regulatory networks might explain the omnigenic nature of complex trait. Disruption of regulatory networks is also a key mechanism underlying human diseases. However, very little is known about trans-regulatory mechanism to date, because detecting trans-regulatory effects is very challenging.
I am very interested and passionate about trans gene regulation! Our team take on the challenge of identifying and understanding trans gene regulation by using statistical/computational and experimental approaches. We developed statistical methods that drastically improved the detection powers of trans genetic effects in gene/protein expression data. With high quality trans-eQTLs, we will be able to study the trans regulatory mechanism in more depth. We are also working on combing CRISPR perturbations, RNA-sequencing, and new statistical tools to identify trans regulation signals.
- Liu et al., Trans effects on gene expression can drive omnigenic inheritance. 2019. Cell
- Liu et al., GBAT: a gene-based association test for robust detection of tans-gene regulation. 2020. Genome Biology
- Wang et al., Trans-eQTL mapping in gene sets identifies network effects of genetic variants. 2022. bioRxiv
- Check out our website http://networks-liulab.org for resources on trans-eQTL and trans gene regulation
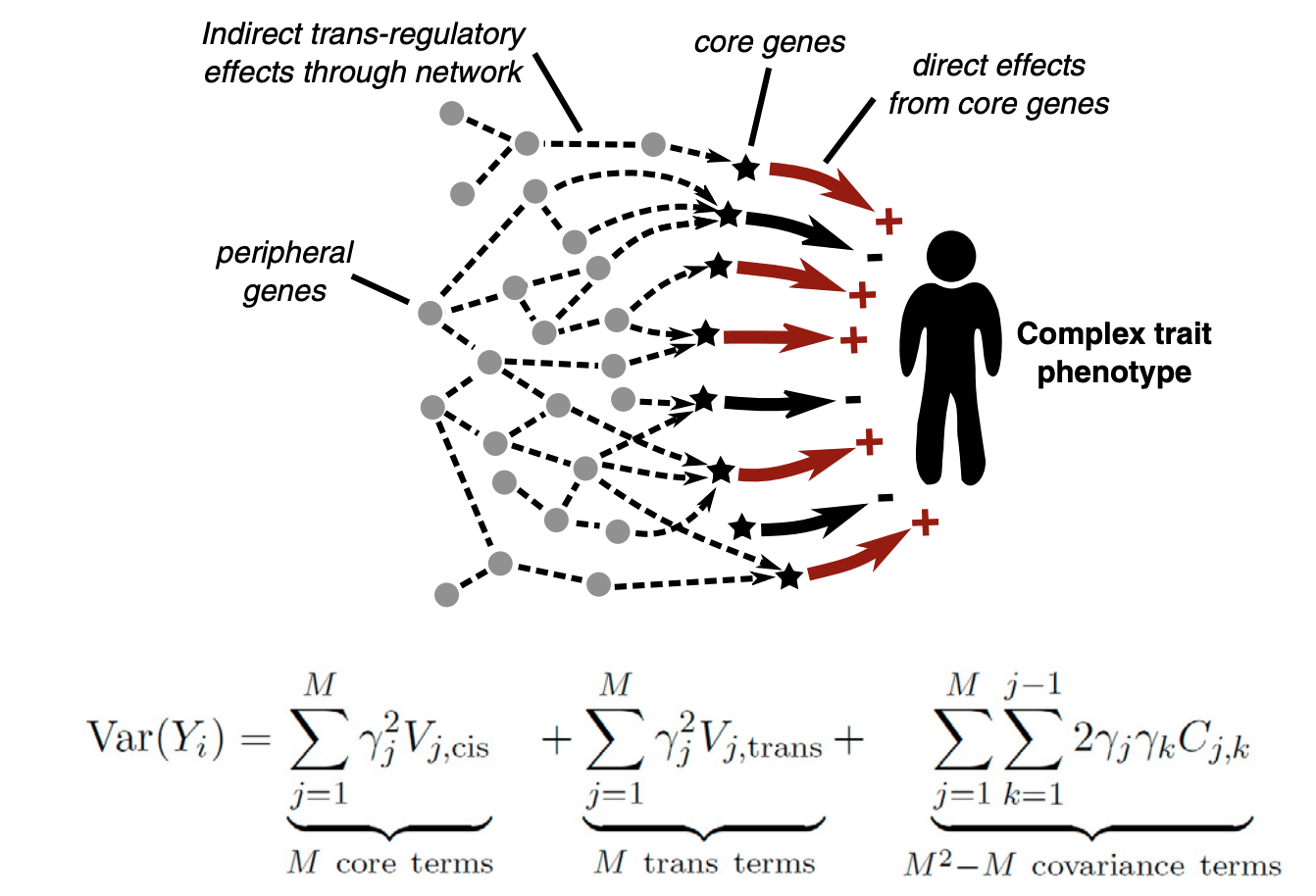
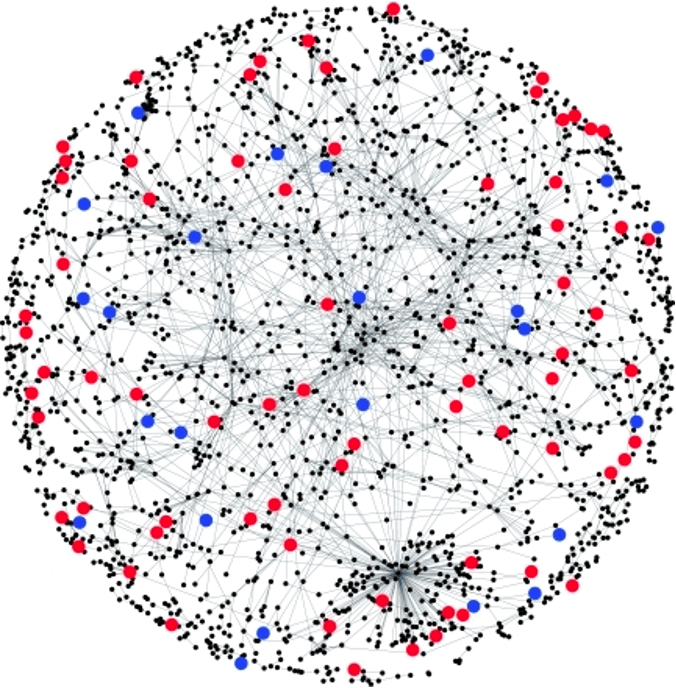
Natural selection and polygenic score (PRS) portability across ancestries
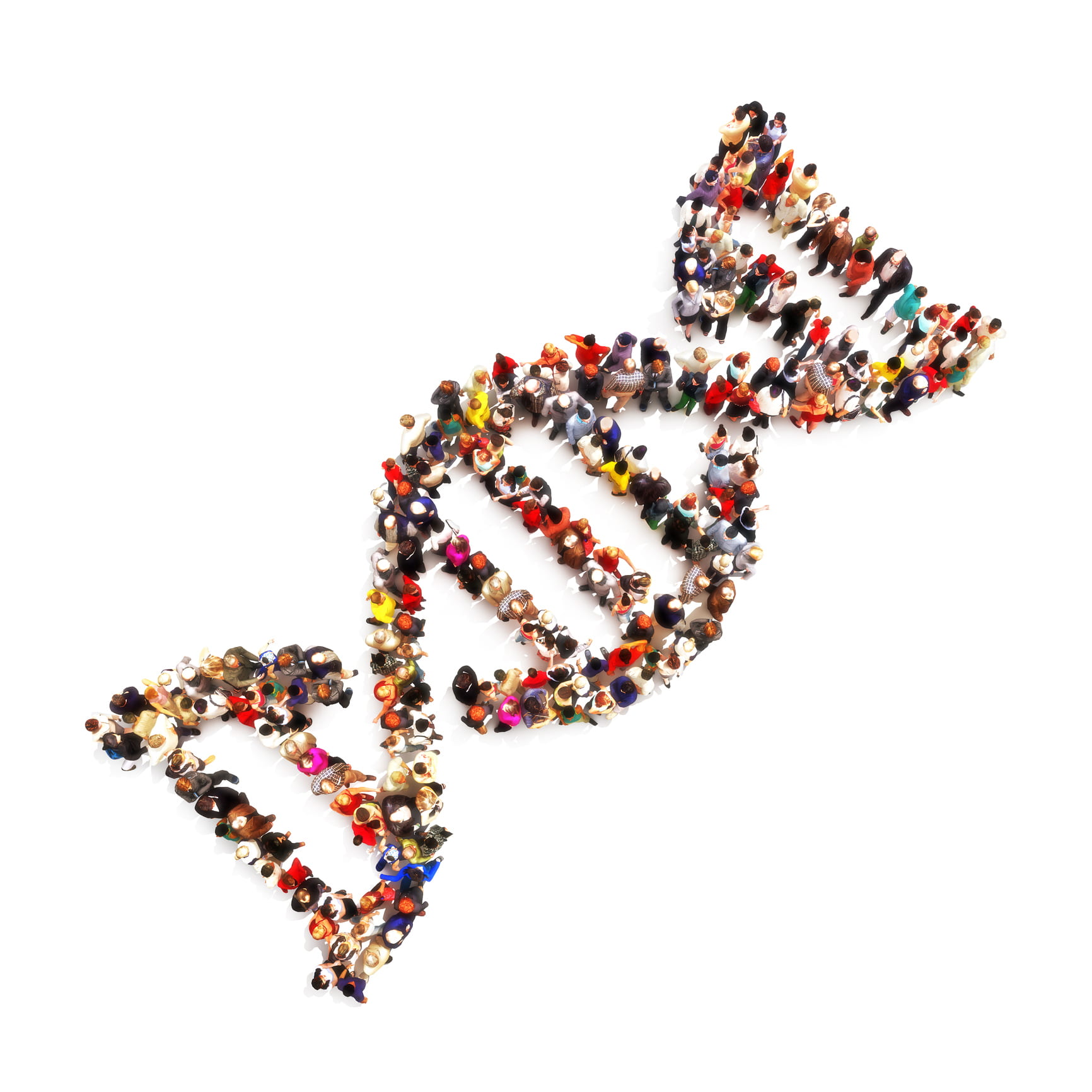
Genome-wide association studies (GWAS) are overwhelmingly biased toward European ancestries. However, how well is the genetic knowledge gained from European GWAS transferrable to individuals of other ancestries? In recent years, nearly all studies agree that prediction accuracy of complex traits in non-European populations is very low. This is known as the low cross-ancestry portability of the polygenic prediction problem, which highlighted the need to understand the sharing and population-specific genetic effects of disease and complex traits.
We answer important questions on the sharing of genetic effects across ancestries and their impact on cross-ancestry genetic prediction. The goal is to understand the factors (such as sharing of genetic effects, allele frequency differences and selection) leading to low portability of PRS and then develop new strategies to improve genetic prediction and prediction portability.